Working on GPU-accelerated data science libraries at NVIDIA, I think about accelerating code through parallelism and concurrency pretty frequently. You might even say I think about it all the time.
In light of that, I recently took a look at some of my old web scraping code across various projects and realized I could have gotten results much faster if I had just made a small change and used Python’s built-in concurrent.futures library. I wasn’t as well versed in concurrency and asynchronous programming back in 2016, so this didn’t even enter my mind. Luckily, times have changed.
In this post, I’ll use concurrent.futures
to make a simple web scraping task 20x faster on my 2015 Macbook Air. I’ll briefly touch on how multithreading is possible here and why it’s better than multiprocessing, but won’t go into detail. This is really just about highlighting how you can do faster web scraping with almost no changes.
Let’s say you wanted to download the HTML for a bunch of stories submitted to Hacker News. It’s pretty easy to do this. I’ll walk through a quick example below.
First, we need get the URLs of all the posts. Since there are 30 per page, we only need a few pages to demonstrate the power of multithreading. requests
and BeautifulSoup
make extracting the URLs easy. Let’s also make sure to sleep
for a bit between calls, to be nice to the Hacker News server. Even though we’re only making 10 requests, it’s good to be nice.
So, we’ve got 289 URLs. That first one sounds pretty cool, actually. A business card that runs Linux?
Let’s download the HTML content for each of them. We can do this by stringing together a couple of simple functions. We’ll start by defining a function to download the HTML from a single URL. Then, we’ll run the download function on a test URL, to see how long it takes to make a GET
request and receive the HTML content.
Right away, there’s a problem. Making the GET
request and receiving the response took about 500 ms, which is pretty concerning if we need to make thousands of these requests. Multiprocessing can’t really solve this for me, as I only have two physical cores on my machine. Scraping thousands of files will still take thousands of seconds.
We’ll solve this problem in a minute. For now, let’s redefine our download_url
function (without the timers) and another function to execute download_url
once per URL. I’ll wrap these into a main
function, which is just standard practice. These functions should be pretty self-explanatory for those familiar with Python. Note that I’m still calling sleep
in between GET
requests even though we’re not hitting the same server on each iteration.
I modified a web-scraping template I use for most of my Python-based scraping needs to fit your needs. Verified it worked with my own login info. The way it works is by mimic-ing a browser and maintaining a cookieJar that stores your user session. Got it to work with BeautifulSoup for you as well.
And, now on the full data.
- In this post, we are going to scrape data from Linkedin using Python and a Web Scraping Tool.We are going to extract Company Name, Website, Industry, Company Size, Number of employees, Headquarters Address, and Specialties.
- In this post, we are going to scrape data from Linkedin using Python and a Web Scraping Tool. We are going to extract Company Name, Website, Industry, Company Size, Number of employees, Headquarters Address, and Specialties.
- # djangowebscrapingexample $ pipenv shell $ django-admin startproject djangowebscrapingexample. $ python manage.py createsuperuser $ python manage.py makemigrations $ python manage.py migrate. Unpacking some of the commands above, we’ll be creating a virtual environment shell instance to execute Django commands.
As expected, this scales pretty poorly. Cinema 4d download mac. On the full 289 files, this scraper took 319.86 seconds. That’s about one file per second. At this point, we’re definitely screwed if we need to scale up and we don’t change our approach.
So, what do we do next? Google “fast web scraping in python”, probably. Unfortunately, the top results are primarily about speeding up web scraping in Python using the built-in multiprocessing
library. This isn’t surprising, as multiprocessing is easy to understand conceptually. But, it’s not really going to help me.
The benefits of multiprocessing are basically capped by the number of cores in the machine, and multiple Python processes come with more overhead than simply using multiple threads. If I were to use multiprocessing on my 2015 Macbook Air, it would at best make my web scraping task just less than 2x faster on my machine (two physical cores, minus the overhead of multiprocessing).
Luckily, there’s a solution. In Python, I/O functionality releases the Global Interpreter Lock (GIL). This means I/O tasks can be executed concurrently across multiple threads in the same process, and that these tasks can happen while other Python bytecode is being interpreted.
Oh, and it’s not just I/O that can release the GIL. You can release the GIL in your own library code, too. This is how data science libraries like cuDF and CuPy can be so fast. You can wrap Python code around blazing fast CUDA code (to take advantage of the GPU) that isn’t bound by the GIL!

While it’s slightly more complicated to understand, multithreading with concurrent.futures
can give us a significant boost here. We can take advantage of multithreading by making a tiny change to our scraper.
Notice how little changed. Instead of looping through story_urls
and calling download_url
, I use the ThreadPoolExecutor
from concurrent.futures
to execute the function across many independent threads. I also don’t want to launch 30 threads for two URLs, so I set threads
to be the smaller of MAX_THREADS
and the number of URLs. These threads operate asynchronously.
That’s all there is to it. Let’s see how big of an impact this tiny change can make. It took about five seconds to download five links before.
Six times faster! And, we’re still sleeping for 0.25 seconds between calls in each thread. Python releases the GIL while sleeping, too.
What about if we scale up to the full 289 stories?
17.8 seconds for 289 stories! That’s way faster. With almost no code changes, we got a roughly 18x speedup. At larger scale, we’d likely see even more potential benefit from multithreading.
Basic web scraping in Python is pretty easy, but it can be time consuming. Multiprocessing looks like the easiest solution if you Google things like “fast web scraping in python”, but it can only do so much. Multithreading with concurrent.futures can speed up web scraping just as easily and usually far more effectively.
Note: This post also syndicated on my Medium page.
Today I would like to do some web scraping of Linkedin job postings, I have twoways to go: - Source code extraction - Using the Linkedin API
I chose the first option, mainly because the API is poorly documented and Iwanted to experiment with BeautifulSoup.BeautifulSoup in few words is a library that parses HTML pages and makes it easyto extract the data.
Official page: BeautifulSoup web page
Now that the functions are defined and libraries are imported, I’ll get jobpostings of linkedin.
The inspection of the source code of the page shows indications where to accesselements we are interested in.
I basically achieved that by ‘inspecting elements’ using the browser.
I will look for “Data scientist” postings. Note that I’ll keep the quotes in mysearch because otherwise I’ll get unrelevant postings containing the words“Data” and “Scientist”.
Below we are only interested to find div element with class ‘results-context’,which contains summary of the search, especially the number of items found.
Now let’s check the number of postings we got on one page
To be able to extract all postings, I need to iterate over the pages, thereforeI will proceed with examining the urls of the different pages to work out thelogic.
url of the first page
Download adobe fonts to mac. https://www.linkedin.com/jobs/search?keywords=Data+Scientist&locationId=fr:0&start=0&count=25&trk=jobs_jserp_pagination_1
second page
https://www.linkedin.com/jobs/search?keywords=Data+Scientist&locationId=fr:0&start=25&count=25&trk=jobs_jserp_pagination_2
third page
https://www.linkedin.com/jobs/search?keywords=Data+Scientist&locationId=fr:0&start=50&count=25&trk=jobs_jserp_pagination_3
there are two elements changing :
- start=25 which is a product of page number and 25
- trk=jobs_jserp_pagination_3
I also noticed that the pagination number doesn’t have to be changed to go tonext page, which means I can change only start value to get the next postings(may be Linkedin developers should do something about it …)
As I mentioned above, all the information about where to find the job detailsare made easy thanks to source code viewing via any browser
Next, it’s time to create the data frame
Now the table is filled with the above columns.
Just to verify, I can check the size of the table to make sure I got all thepostings
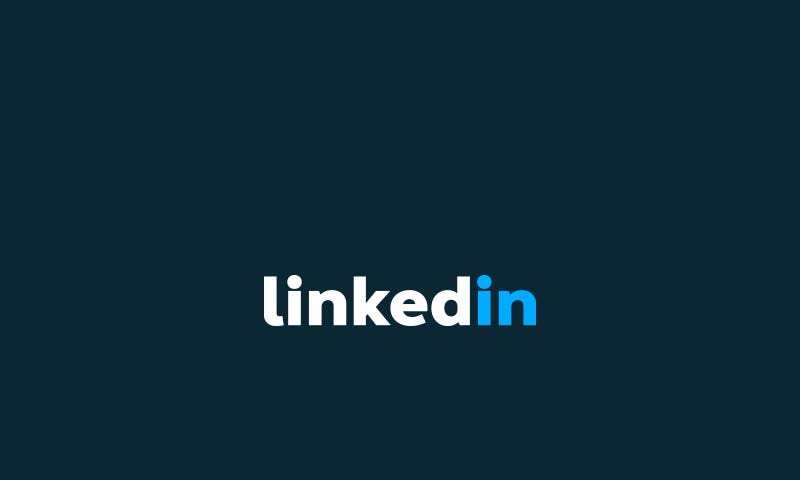
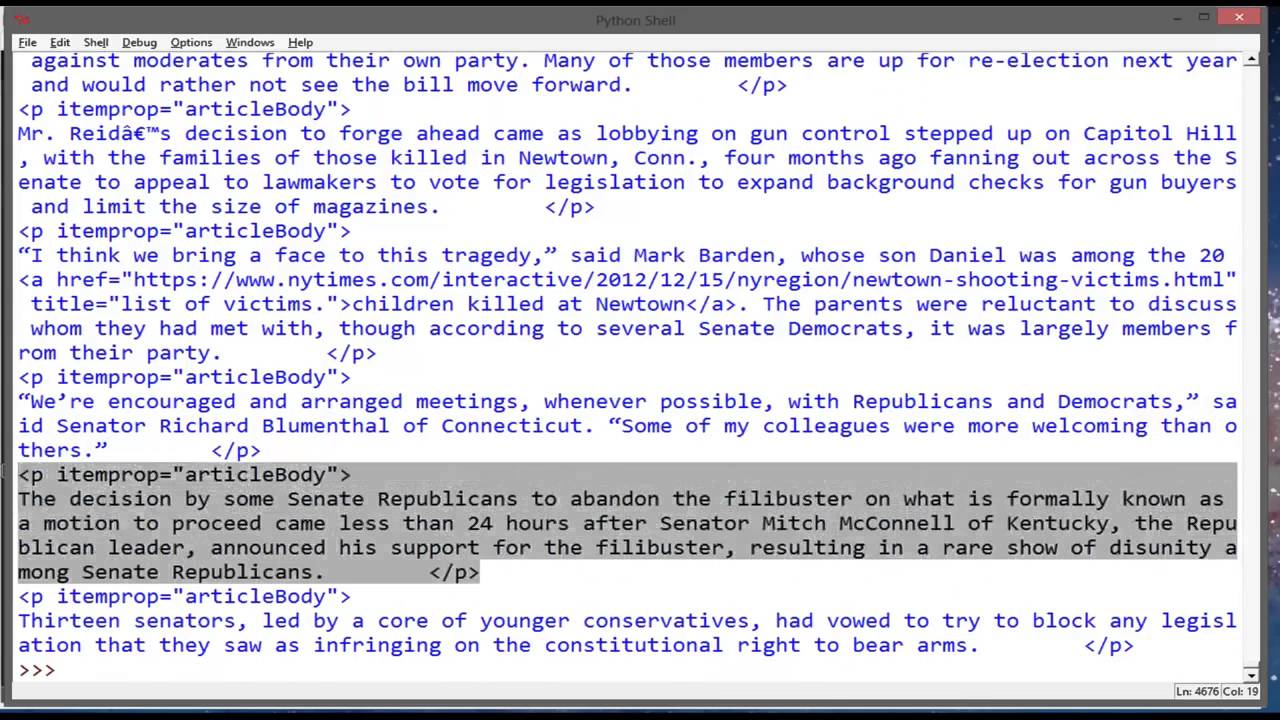
In the end, I got an actual dataset just by scraping web pages. Gathering datanever have been as easy.I can even go further by parsing the description of each posting page andextract information like:
- Level
- Description
- Technologies
…
There are no limits to which extent we can exploit the information in HTML pagesthanks to BeautifulSoup, you just have to read the documentation which is verygood by the way, and get to practice on real pages.
Web Scraping Linkedin Python Interview
Ciao!
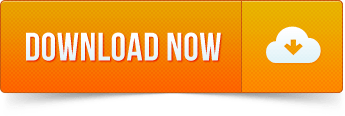